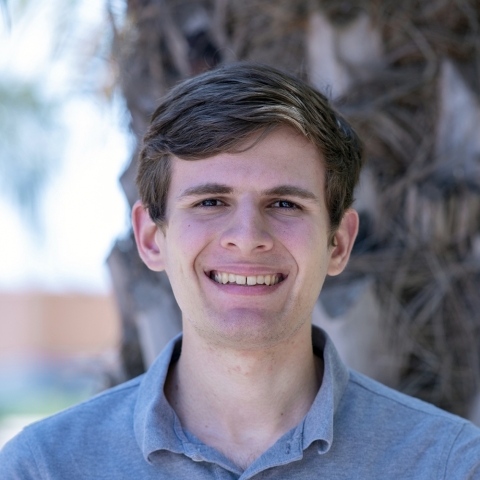
Date:
Location:
Speaker:
Title: Tackling fundamental multiscale modeling problems with relative entropy coarse-graining
Abstract
Many chemical, material, and biological systems of interest to scientific and engineering applications involve inherently multiscale phenomena and processes. Application of molecular modeling and simulation methods to these systems promises to provide fundamental understanding of their physics and predict their behavior under various conditions. However, these techniques have specific limited ranges of length and time scales over which they can be practically applied, necessitating multiscale modeling approaches. For instance, bottom-up coarse-graining allows simplified molecular models to be systematically constructed from more detailed atomistic reference systems, and used for simulations over length and time scales that would otherwise be prohibitively computationally expensive. Although it offers a route to models ostensibly free from ad hoc approximations or requirements for empirical data, a few important problems remain in its use, including achieving representability (the ability of coarse models to predict desired properties of atomistic systems) and transferability (their ability to do so over a range of thermodynamic conditions or chemical identities).
This work, using the relative entropy coarse-graining approach, addresses these fundamental problems in a few classes of systems. We present a method for systematically building temperature transferable models by encoding energy fluctuations normally lost in coarse-graining back into these models in a thermodynamically consistent manner, and demonstrate its use on several molecular fluid systems. We present and validate an approach for developing coarse-grained models of peptides using multiple optimized reference ensembles. These models are predictive of peptide chain conformational properties, secondary structure, and amyloid aggregation propensity. We use this approach to create a model tailored to the aggregation-prone PHF6 peptide (found in the Alzheimer’s disease-implicated tau protein) enabling large-scale aggregation simulations, mapping of the conformational and free energetic landscape of PHF6 oligomers, and exploration of the relationship between the properties of anionic polyelectrolytes and their ability to induce PHF6 aggregation. These applications all show the power of bottom-up coarse-graining to produce predictive, transferable models that can be used to study complex phenomena out of the practical reach of ordinary atomistic simulations.