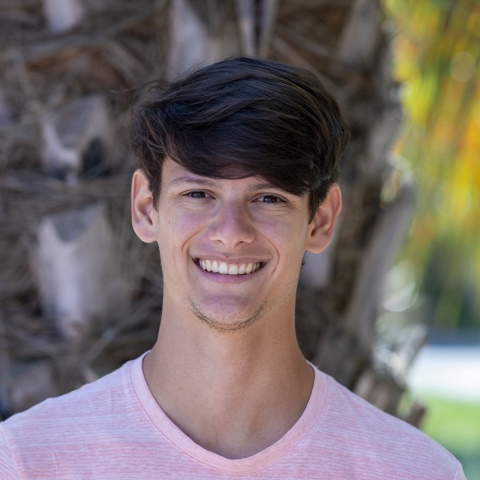
Date:
Location:
Speaker:
Title: Towards a Turnkey Model Predictive Controller: Identification, Application, and Theory
Abstract
An out-of-the-box model predictive control (MPC) algorithm, or a "turnkey" model predictive controller has long been a dream of both academics and practitioners. MPC practice currently includes time-consuming and ad hoc tuning steps to achieve adequate performance in the face of persistent disturbances and plant-model mismatch. In this dissertation, we present progress towards developing a turnkey model predictive controller by developing identification methods suitable for out-of-the-box MPC implementations, applying those identification methods to the offset-free control of real-world systems, and developing the theory of the stability of MPC under plant-model mismatch.
A unifying theme of this work is the use of integrating disturbances in MPC to achieve offset-free performance. We first propose algorithms for identifying models with integrating disturbances. Standard identification methods are augmented with disturbance models to provide a straightforward path towards data-based MPC designs. Maximum likelihood identification is rigorously applied with eigenvalue constraints to ensure closed-loop stability of the offset-free MPC design. Next, we apply identification methods to the offset-free control of two real-world systems: a benchmark temperature controller (TCLab), and an industrial-scale chemical reactor. Both case studies showcase the ability of the identification algorithms to produce models adequate for out-of-the-box MPC designs with guaranteed offset-free performance. The industrial application also demonstrates an outsize real-world benefit for adopting a turnkey approach, where we report a 38% improvement in setpoint tracking performance compared to an existing hand-tuned controller. Last, we present the first results on the stability of nonlinear offset-free MPC, with and without plant-model mismatch.